Welcome! The TBD pedestrian dataset is a dataset primarily collected in pedestrian-rich environments. Our dataset highlights the following components: human-verified labels grounded in the metric space, a combination of top-down and ego-centric views, and naturalistic human behavior in the presence of a socially appropriate “robot”.
Dataset Highlights
Human verified metric space labels:
Having labels grounded in metric space eliminates the possibility that camera poses might have an effect on the scale of the labels. It also makes the dataset useful for robot navigation related research because robots plan in the metric space rather than image space.
Top-down and ego-centric views:
A dataset that contains both top-down views and ego-centric views will be useful for research projects that rely on ego-centric views. This allows ego-centric inputs to their models, while still having access to ground truth knowledge of the entire scene.
Naturalistic human behavior with the presence of a “robot”:
The “robot” that provides ego-centric view data collection is a cart being pushed by human. Doing so reduces the novelty effects from the surrounding pedestrians. Having the “robot” being pushed by humans also ensures safety for the pedestrians and its own motion has more natural human behavior. As such, the pedestrians also react naturally around the robot by treating it as another human agent.
Example Pedestrian Interactions
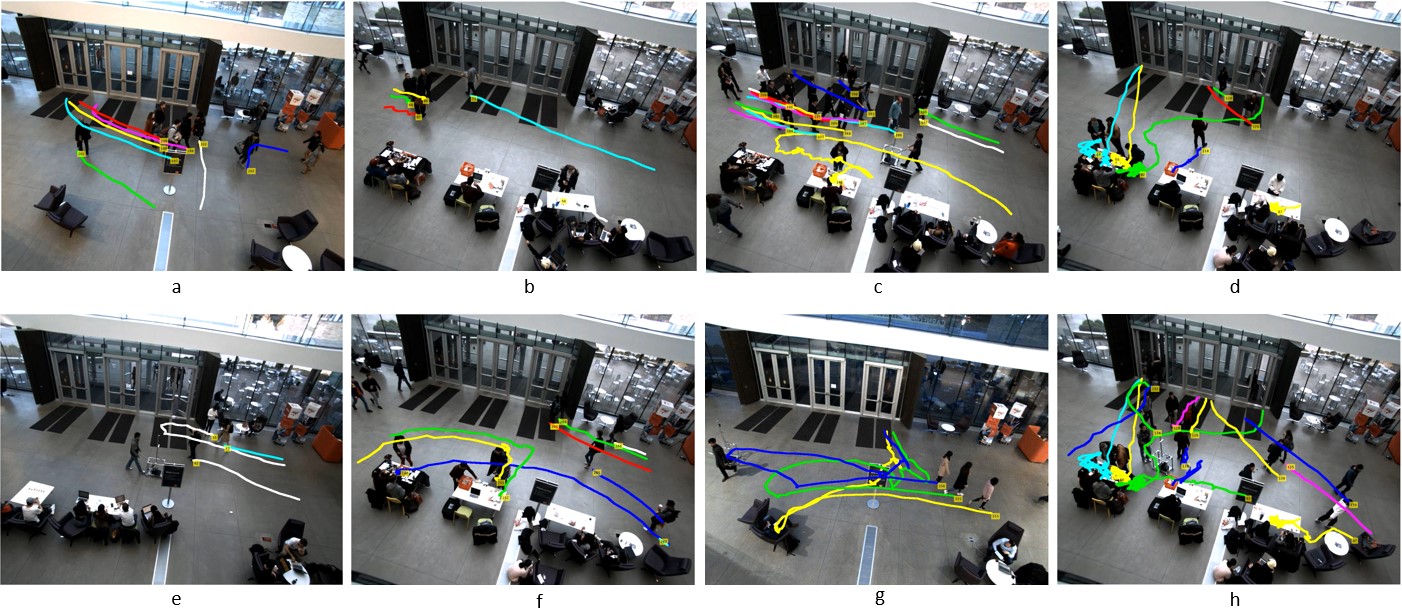
Downloads
The button below will take you to our datasets on KiltHub. Descriptions of the data organization and label files are in the readme file. Set 2 is the larger and newer dataset.
If you are a member of the Robotics Institute or Carnegie Mellon, please contact Aaron Steinfeld for local access options.
This effort is IRB approved under Carnegie Mellon University protocols STUDY2019_00000116 and STUDY2021_00000199. Please contact Aaron Steinfeld for IRB related issues.
Hardware – Set 2
Set 2 is the recommended set to use. It is larger and contains 3D LiDAR data. The robotic suitcase has better localization capability, so the label synchronization between the top-down view and the ego-centric view is very accurate.
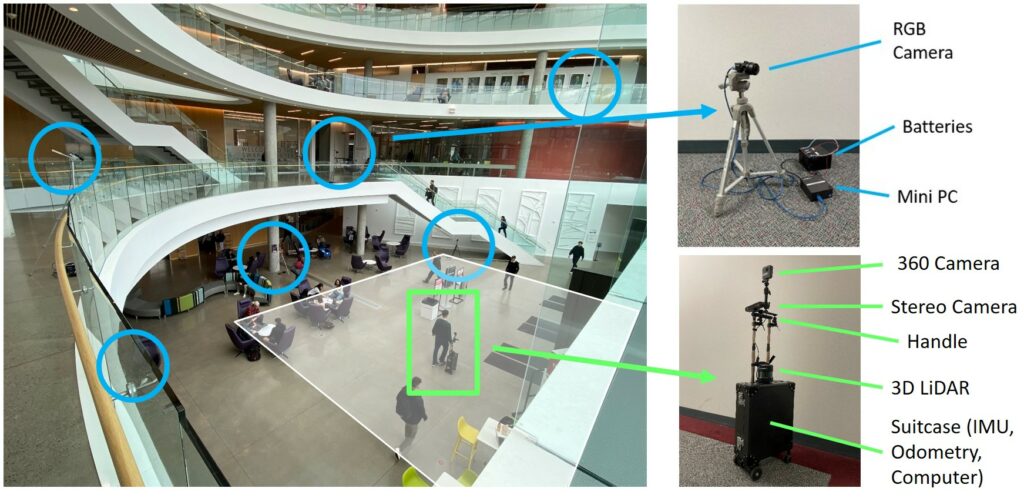
We positioned three FLIR Blackfly RGB cameras surrounding the scene on the upper floors overlooking the ground level at roughly 90 degrees apart from each other. Compared to a single overhead camera, multiple cameras ensure better pedestrian labeling accuracy. The RGB cameras are connected to portable computers powered by lead-acid batteries. We also positioned three more units on the ground floor but did not use them for pedestrian labeling.
In addition, we pulled a robotic suitcase side-by-side through the scene. The robotic suitcase is a converted carry-on rolling suitcase. It is equipped with an IMU and a 3D LiDAR sensor. The robot’s computer, batteries, and all its internal components are hidden inside the suitcase, so pushing the robot resembles pushing a suitcase. We selected this robot because of its inconspicuous design to reduce curious, unnatural reactions from nearby pedestrians, as curious pedestrians may intentionally block robots or display other unnatural movements. In addition, a ZED stereo camera was mounted to collect both ego-centric RGB views and depth information about the scene. A GoPro Fusion 360 camera for capturing high-definition 360 videos of nearby pedestrians was mounted above the ZED. Data from the onboard cameras are useful in capturing pedestrian pose data and facial expressions (annotation in progress).
Hardware – Set 1
Set 1 is an older version of our dataset. It is smaller and the synchronization between the top-down view and the ego-centric view is less accurate, but it still contains interesting pedestrian interaction data. It can be used to complement set 2.
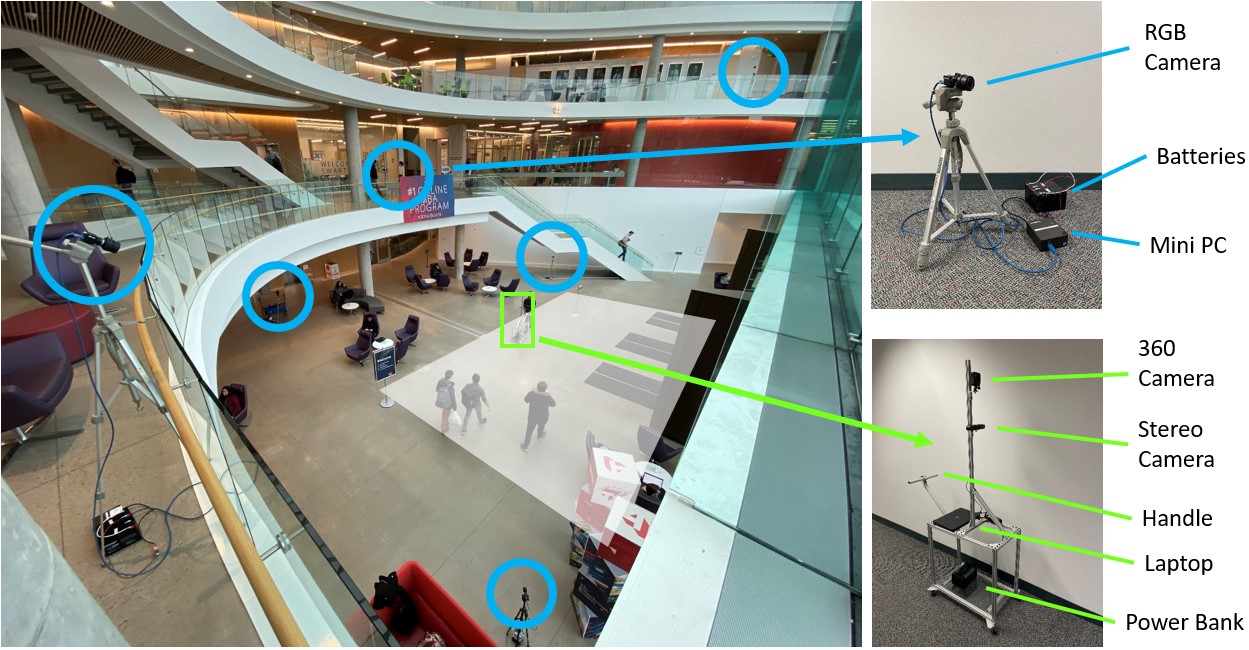
Instead of the suitcase robot, we pushed a cart through the scene, which was equipped with the same ZED stereo camera. The same GoPro Fusion 360 camera was mounted above the ZED at human chest level. The ZED camera was powered by a laptop with a power bank.
Statistics
Due to the larger size, Set 2 is the recommended set to use.
Set | Duration (min) | Duration w Robot/Cart (min) | # Of Pedestrians | Label Frequency (Hz) |
2 | 626 | 213 | 10300 | 10 |
1 | 133 | 51 | 1416 | 60 |
Publication
Set 2 – Wang, A., Sato, D., Corzo, Y., Simkin, S., & Steinfeld, A. (2024). TBD Pedestrian Data Collection: Towards Rich, Portable, and Large-Scale Natural Pedestrian Data. 2024 IEEE International Conference on Robotics and Automation. IEEE, 2024. [link]
Set 1 – Wang, A., Biswas, A., Admoni, H., & Steinfeld, A. (2022). Towards Rich, Portable, and Large-Scale Pedestrian Data Collection. ArXiv, abs/2203.01974. [link]
Acknowledgments
This project was supported by grants (IIS-1734361 and IIS-1900821) from the National Science Foundation. Additional support was provided by the Office of Naval Research (N00014-18-1-2503) and the National Institute on Disability, Independent Living, and Rehabilitation Research (NIDILRR grant number 90DPGE0003).